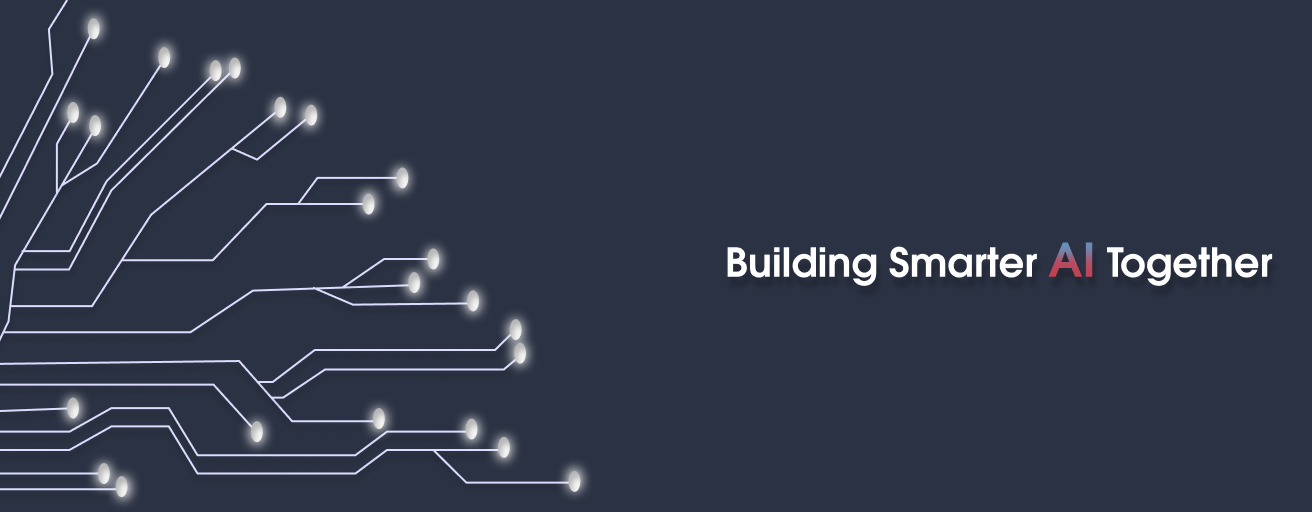
Healthcare data collection is at the forefront of transforming patient outcomes, optimizing hospital operations, and accelerating advancements in medical science. But how is this data collected, managed, and used effectively? This blog will explore the importance of healthcare data collection, tackle its challenges, examine the technologies involved, and provide you with actionable best practices.
Whether you're a healthcare professional, data scientist, or health administrator, this post will serve as a comprehensive guide for understanding and navigating the complexities of healthcare data collection.
Why is Healthcare Data Collection Important?
Healthcare data collection underpins nearly every aspect of modern healthcare. It enables institutions to make data-driven decisions that lead to improved patient care, efficient processes, and innovative medical developments.
Here’s why it’s critical:
Improving Patient Outcomes: Comprehensive data allows for better disease management, early diagnoses, and personalized treatment options.
Operational Efficiency: Data optimizes resource allocation, staffing, inventory management, and financial planning.
Fueling Research and AI Development: Collected data is often the foundation for training AI/ML (artificial intelligence and machine learning) models that enhance automation and predictive analytics in healthcare.
Without accurate and consistent data collection practices, the healthcare sector would struggle to meet its most pressing challenges.
Types of Healthcare Data Collected
Healthcare is a data-rich field, collecting diverse information ranging from clinical to operational. Understanding these data types paints a clearer picture of what is being collected and why.
Clinical Data
Includes patient records, lab results, imaging data, and prescriptions.
Used for diagnosing conditions, planning treatment, and monitoring outcomes.
Operational Data
Encompasses hospital admissions, discharge records, staffing schedules, and inventory management.
Improves efficiency and ensures healthcare institutions run smoothly.
Patient-Generated Data
Data collected via wearables, mobile health apps, or patient surveys.
Supports preventive care and helps patients take an active role in managing their health.
Financial Data
Includes billing information, insurance claims, and cost reports.
Useful for assessing financial performance and creating sustainable healthcare policies.
Public Health Data
Large-scale health reports that inform policymakers about disease trends, outbreaks, or community health standards.
Essential for drafting public health policies and preventive strategies.
Challenges in Healthcare Data Collection
Despite its importance, healthcare data collection comes with a unique set of challenges:
1. Data Silos
Healthcare organizations often manage data across different systems, creating silos that restrict seamless communication and sharing.
2. Privacy Concerns
Collecting sensitive patient data requires rigorous compliance with regulations like HIPAA (Health Insurance Portability and Accountability Act) to protect privacy.
3. Data Quality Issues
Incomplete, outdated, or incorrect data can hinder decision-making and skew research outcomes.
4. High Costs
Implementing robust data collection and management systems can be resource-intensive, especially for smaller healthcare facilities.
5. Interoperability
Differing formats and systems used by various healthcare providers hinder the efficient flow of data.
Technologies Used in Healthcare Data Collection
Several technologies are revolutionizing how healthcare data is collected and managed:
1. Electronic Health Records (EHRs)
EHRs digitize patient records, making data more accessible, shareable, and manageable. Platforms like Epic and Cerner are leading players in this space.
2. Wearable Technology and IoT Devices
Smartwatches, fitness trackers, and implantable devices collect real-time health data like heart rate, blood sugar levels, and activity metrics.
3. Artificial Intelligence (AI) and Machine Learning (ML)
Companies like Macgence specialize in providing healthcare data to train AI/ML models. These models enable predictive analytics, automate tasks, and improve diagnostic accuracy.
4. Cloud Computing
Cloud infrastructure like AWS HealthLake or Google Cloud Healthcare API stores vast volumes of data securely while making it easily accessible to authorized users.
5. Blockchain Technology
This emerging technology ensures the secure, transparent, and traceable exchange of medical data.
Best Practices for Healthcare Data Collection
Implementing disciplined practices can help organizations overcome challenges and maximize the potential of healthcare data collection.
Prioritize Data Privacy and Compliance
Follow regulatory requirements, such as HIPAA and GDPR, to ensure patient trust and avoid fines.
Implement encryption, firewalls, and secure access controls.
Invest in Interoperable Systems
Opt for software solutions that integrate smoothly with other systems, facilitating seamless data exchange.
Standardize Data Formats
Use standardized coding systems like ICD (International Classification of Diseases) or HL7 FHIR (Fast Healthcare Interoperability Resources) to ensure consistency.
Leverage AI/ML for Data Analysis
Platforms like Macgence can provide high-quality, annotated data to train AI/ML models for better data insights.
Monitor Data Quality Regularly
Regular audits can help identify gaps and ensure that stored data is accurate, relevant, and up-to-date.
Train Your Staff
Educate healthcare professionals about the importance of meticulous data entry and compliance.
Future Trends in Healthcare Data Collection
The landscape of healthcare data collection continues to evolve rapidly, with several exciting trends on the horizon.
Personalized Medicine
Advances in genetic profiling and AI will allow healthcare providers to design precise treatments based on individual genetic compatibility.
Integration of Telehealth Data
The rise of telemedicine means more patient data will be collected remotely, requiring secure and efficient integration systems.
Expansion of Predictive Analytics
Predictive analytics, powered by machine learning, will offer unparalleled insights into patient care, operational improvements, and disease prevention.
Edge Computing for Real-Time Monitoring
Devices with edge computing capabilities will process healthcare data closer to its collection source, enabling real-time decision-making.
Global Data Collaboration
Expect to see more cross-border collaboration in data sharing for global health initiatives like pandemic tracking and vaccination programs.
Make Data-Driven Healthcare a Reality
Healthcare data collection represents a tremendous opportunity for organizations to revolutionize patient care and operational efficiency. However, it’s essential to invest in the right technologies and practices to maximize value from the data.
If you're looking to leverage high-quality data for training AI/ML models, companies like Macgence are at the forefront of providing annotated healthcare data. Whether you're a data scientist or health administrator, Macgence can help make your next AI project a success.
Want to explore more about industry-leading data solutions for AI? Contact Macgence today and take the first step towards smarter healthcare.
Write a comment ...